Modern technologies can help companies make profitable use of the large quantities of data produced by the advancement of digitalization. The key is artificial intelligence (AI).
Artificial intelligence: using big data profitably
Thanks to tremendous breakthroughs in research, unstructured data such as images, text, spoken language and audio files can now also be processed and analyzed. Artificial intelligence supports people without making human work redundant. Yet the implementation of such processes requires expert knowledge and experience.
Lufthansa Industry Solutions offers a wide-ranging portfolio in this field to help companies work more efficiently and prepare for the future not only by automating and optimizing processes, but even by enabling entirely new digital business models. In the future, all industries will be able to benefit from the possibilities offered by artificial intelligence (AI) by using computer vision to identify defects, natural language processing to scan documents and texts, and audio analysis to support quality assurance.
How do companies stand to benefit from artificial intelligence?
From logistics to invoice auditing and production, the use of data and its further processing as part of intelligent systems has the potential to save resources while increasing the quality of products and the efficiency of processes.
Data analytics, ML and AI: the path from data to application
Those considering the use of artificial intelligence may be familiar with terms such as “data analytics” and “machine learning (ML).” But what do these things all have in common? It is often impossible to draw a clear line between data analytics and AI: Both disciplines are closely intermeshed and, in many cases, even build on one another. What is more, both involve the efficient processing of large quantities of data. The insights gained through data analysis can often help to create AI.
The aim of data analytics is to glean insights from large volumes of data. Data analytics combines knowledge of the respective field (known as domain knowledge) with an analytical approach specifically tailored to each data set. The insights gained are then evaluated by human experts.
Machine learning is a discipline of AI that involves developing processes and algorithms that analyze and universalize patterns in data in order to derive models for predictions or automated decisions, to name just a few examples. Machine learning focuses on algorithms and methods, often without explicitly adding domain knowledge.
AI can be understood to mean a system that supports decisions or makes them autonomously. Today, AI is usually trained with data using ML and can be used consistently in new situations. Human interpretations of findings and results, as in data analytics, usually do not play a role in AI.
Artificial intelligence: possibilities and limitations
As with any technology, AI is associated with both advantages and disadvantages. While AI applications offer tremendous potential for enhancing quality, their development and operation also present business with challenges. Data quality and quantity are essential, since today’s AI is usually trained with data. Although AI does not need to be sophisticated, the necessary level of complexity may rise depending on the task – something that often has to do with the problem domain. The higher the number of parameters to be considered, the more computation time and memory is necessary. The success of an AI project may therefore also depend on the IT resources available. Along with access to expertise, the availability of these IT resources must be determined in advance.
According to a 2019 study by Lünendonk entitled “Artificial intelligence: a study on the status quo at German companies and in future applications” [“Künstliche Intelligenz. Eine Studie zum Status quo in deutschen Unternehmen und zu zukünftigen Anwendungsfällen”], 59 percent of companies believe they lack in-house AI expertise. At 71 percent, however, the greatest challenge lies in data quality and/or availability.
Today, most approaches involve developing AI only to address certain issues or problems. The ML algorithms do not take potential knowledge outside this narrowly defined range into consideration. Human intelligence can compensate for this shortcoming and can be explicitly used in the creation of models during the development of AI. The same goes for ethical considerations. Wherever automated decisions are made without human input, potential consequences must be examined and taken into account accordingly during data gathering and processing. Ultimately, AI is only capable of learning what it receives in the form of data. As a result, AI may continue to perpetuate unjustified assumptions or errors contained in said data. This aspect must be addressed during the development of AI.
AI: sources of errors
Erroneous model assumptions may cause an AI system to learn patterns that do not reflect the true relationships between cause and effect
Solution: AI experts work with domain specialists to test models and parameters syntactically and semantically.
Omnipresent computational power may result in overly complex AI setups. Such AI systems are more likely to memorize data verbatim rather than making generalized assumptions.
Solution: It is essential to understand the significance of model assumptions and hyperparameters so as to make a reasonable and appropriate selection. Well-trained AI experts need to be capable of doing just that.
A domain-agnostic approach is taken to raw data.
Solution: Domain specialists and AI experts prepare existing data in accordance with semantic and model-specific criteria.
What should good artificial intelligence for businesses be able to do?
A wide range of criteria needs to be met for AI to make full use of its benefits. Good AI
- provides people with seamless support and performs monotonous tasks,
- independently identifies and universalizes patterns in training data so it can also handle new data streams correctly,
- is robust and able to cope with data stream variations,
- can react appropriately to changes in the data stream and adjust results accordingly, and
- reaches reasonable and justifiable decisions.
During the development of AI, it is essential to
- address and economically optimize business cases,
- minimize bottlenecks in existing business processes,
- take scalability and elasticity into consideration,
- define operating concepts, as AI presents new challenges for IT departments and service providers once in operation, and
- calculate operating costs for various models.
How does artificial intelligence learn?
AI is supposed to learn principles and identify patterns in data. This data should be representative; the raw data should contain all information required to produce the desired results. To simplify an AI system’s learning process, easily computable mathematical features of data points are often used as a substitute for raw data. While these features are currently determined by data scientists and experts in most cases, the AI is often capable of calculating them independently.
It is also possible to create an AI without using historical data. By applying their specialist knowledge, experts can develop and configure AI directly.
As well as the input data, the learning dataset also contains information on the expected outcome. A learning algorithm can thereby compute what errors an AI system would make when processing this data and thereby adjust the model used by the AI to minimize these errors.
If no information or only limited details of the expected outcome are available, learning often takes place on the basis of assumptions or requirements. For example, one might assume that similar data will form a group. New data points can then be allocated to one of these groups on the basis of their similarities. Clustering procedures are based on this assumption. If the general assumption is that the data stream will display regularities, an AI setup can then recognize anomalies – without having previously observed them in the training data.
If an AI system is to make decisions that can be evaluated during learning – in a separate training phase or even in live operation – the reinforcement learning approach is often applied. In this case, the AI learns entire decision-making strategies to interact with its environment. This type of AI can be trained either in live operation or through simulations. For example, it can learn to play board games, control robots or personalize the sales price in an e-commerce shop. At this point, a familiar problem becomes particularly clear: balancing further exploration of the environment to improve the decision-making strategies learned to date against using what has been learned to date. This conundrum is often referred to as “exploration vs. exploitation.”
Objectives and potential applications of AI
Digitalization, data analytics, the Internet of Things (IoT) and machine learning have created tremendous potential for companies in every sector over the past few years. They have made it possible to increase the efficiency of processes while personalizing products, automating customer interaction through chatbots and simplifying B2B communication. Companies with B2C business models have played a pioneering role in this field. Yet there is still tremendous potential, especially in the B2B sector. Examples include logistics processes and combining the IoT and AI to detect errors or perform predictive maintenance. Today, no company is capable of doing business without digital support. AI can be used profitably and beneficially to address all of these aspects.
The goal is to implement an approach to artificial intelligence that is capable of supporting human labor and making it easier in a certain field. Potential applications of artificial intelligence include:
- Early detection of potential defects in machinery based on data from IoT devices to avoid unscheduled maintenance and repair work (predictive maintenance)
- Detecting damage (to freight, for example)
- Forwarding e-mails
- Classifying written customer feedback
- Digitalizing printed invoices and cross-checking invoice information
- Recognizing objects in images
- Analyzing company data
- Analyzing the customer base
- Supporting price management
The freedom achieved by relieving staff of certain routine duties allows them to instead focus on more complex tasks.
Artificial intelligence: Our service portfolio
We develop AIs to support people and their work, and for applications in industry that no human being is capable of performing.
The starting point for this support is always your specific situation and existing digital strategy, which we work together to enhance in order to achieve your vision. Our focus on business cases enables us to implement AI at suitable junctures and avoid technological overcomplication and gimmickry. Tailored AI solutions developed by us – or proven, industry-specific AI solutions that have been configured and adapted by us – can be integrated seamlessly into existing infrastructure and rely on cost-efficient solutions. Wherever data for process optimization is still lacking, we work with our IoT team to develop appropriate solutions or allow you to benefit from our existing solutions for predictive maintenance, error detection, localization and much more.

AI Services from LHIND
We don't just develop completely new AI applications for very individual business needs. In addition, with our AI as a Service solutions, we also offer ready-to-use tools for various use cases - from customer feedback processing to automated email categorization/response or forecasting to AI cash office solutions.
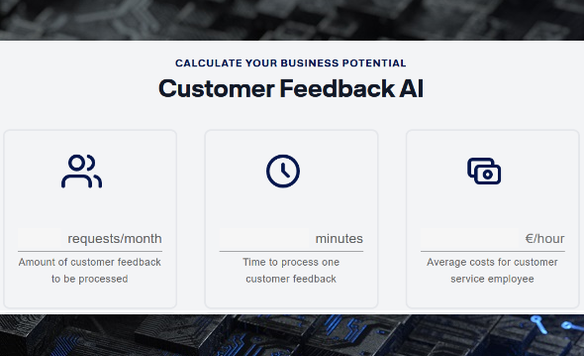
AIaaS Calculator from LHIND
With AIaaS solutions, you can quickly and easily exploit the potential of artificial intelligence for your company and significantly reduce your costs. Use our AIaaS Calculator to calculate the savings you can generate with AI Services, for example in the area of customer feedback processing.
Areas of AI
Image processing science takes images as a starting point and uses them to identify objects, measure sizes, evaluate user inputs and even draw conclusions regarding meanings in a given context.
Examples: Detecting damage to vehicles, performing QA checks on materials and production goods, sorting general cargo, identifying objects in chaotic or confusing situations and evaluating user-generated data
Continuous data streams from sensors (such as audio data, vibration levels or temperatures) are analyzed to identify patterns or deviations. Incoming signals are converted according to the specific application’s requirements in order to facilitate efficient processing.
Examples: Identifying anomalies in case of malfunctions, filtering background noise and intelligent smart home applications
Documents, e-mails, comments and reviews in text form can be understood in a given context and classified accordingly to enable an appropriate response. Beyond the explicit information in the text itself, this process takes into account the implicit context and intention.
Examples: Performing sentiment analysis of social media posts, classifying user comments, analyzing the similarities between different documents and paraphrasing documents.
Our AI experts work hand in hand with domain specialists from our industry-specific departments and continuously discuss and exchange information and ideas in order to create innovative solutions. This allows us to create synergies between solutions from different sectors.
We support companies from the initial analysis and consultation all the way through to training, installation and configuration. Later, we remain available for questions, feedback and fine-tuning. We develop individual solutions that are tailored to our customers’ problems, data sources and specific requirements.
What is Artificial Intelligence? What should a good AI be able to do? Where are their limits? How does an AI learn? And various other aspects are covered in our brochure on Artificial Intelligence. Download the brochure here.
Guidelines on the use of artificial intelligence in its IT projects
Lufthansa Industry Solutions follows the ethical guidelines of the European Commission in the development and use of artificial intelligence in practice. According to these guidelines, a trustworthy AI is characterized by three components that should be fulfilled throughout the entire life cycle of the system:
- it should be lawful and thus comply with all applicable laws and regulations
- it should be ethical and thus guarantee compliance with ethical principles and values, and
- It should be robust, both technically and socially.
AI systems should
- act on justice and common regulations.
- accord to human values, rights and solidarity.
- be beneficial to humanity & environment.
- ease human’s lives but not eliminate human supervision.
- be understandable.
- have algorithmic accountability.
- treat all people fairly and be non-discrimating.
- empower everyone and engage people.
- be secure and respect privacy.
- perform reliably and safely.
- We evaluate existing processes and identify bottlenecks that AI could resolve. This makes it possible to support people in recurrent tasks.
- Our solutions combine continual updates, high-quality AI forecasting or decision-making, and professional operationalization.
- We develop strategies to monitor operationalized AI at all times, as well as strategies to improve AI, adapt it to new framework conditions and ensure all decisions can be fully audited.
- We use established components and supplement these with in-house developments where necessary, taking the latest research developments into account.
- Thanks to the scientific backgrounds of our AI experts, we understand the models and algorithms we use in detail and select them where it is sensible and appropriate to do so.
- We play an active role in scientific discourse and translate the results of research into innovative solutions for industry.
- You benefit from our interdisciplinary expertise using cutting-edge technologies from our partners. In doing so, we take your preferences into account and also incorporate project-specific and legal requirements.
Lufthansa Industry Solutions was recognized as a relevant player in the digital factory in the three use case clusters “Predictive Analytics & Maintenance”, “Traceability” and “Asset & Plant Performance Monitoring” by teknowlogy. According to teknowlogy, the leading independent European market analysis and consulting company for the IT industry, LHIND has proven to be able to address all related use cases in these categories.
Find out more about the topics that matter for your sector
Want to learn more about the IT topics that matter to you? We can keep you up to date with the news from your sector on central issues such as Big Data, Industry 4.0, collaboration platforms and much more besides. We also offer personalized consultations – simply get in touch.